Book Downloads Hub Reads Ebooks Online eBook Librarys Digital Books Store Download Book Pdfs Bookworm Downloads Free Books Downloads Epub Book Collection Pdf Book Vault Read and Download Books Open Source Book Library Best Book Downloads Susannah Calloway Joshua Lane Harry Spiller Gilbert Gottlieb Mark Horrell Richard Iorio Ii Tony Nester Lawrence S Aft
Do you want to contribute by writing guest posts on this blog?
Please contact us and send us a resume of previous articles that you have written.
Modeling With Stochastic Programming Springer In Operations Research And

Stochastic programming is a powerful tool that allows decision-makers to model and solve problems in uncertain environments. By incorporating randomness and uncertainty into mathematical models, stochastic programming provides a framework for optimal decision-making under risk.
Springer is a leading publisher in the field of operations research and offers a wide range of resources for learning and applying stochastic programming techniques. In this article, we will explore the fundamentals of modeling with stochastic programming and discuss the benefits of utilizing Springer's publications in operations research.
What is Stochastic Programming?
Stochastic programming is an extension of traditional mathematical programming that incorporates random variables and uncertainty into its models. It aims to find optimal decisions that not only minimize costs, maximize profits, or achieve other objectives but also account for the inherent risks and uncertainties in the problem at hand.
4 out of 5
Language | : | English |
File size | : | 2827 KB |
Text-to-Speech | : | Enabled |
Screen Reader | : | Supported |
Enhanced typesetting | : | Enabled |
Print length | : | 97 pages |
Lending | : | Enabled |
Unlike deterministic models that assume perfect knowledge of input parameters, stochastic programming allows decision-makers to consider various possible outcomes and their associated probabilities. By explicitly accounting for uncertainty, stochastic programming provides a more robust and realistic approach to decision-making.
Why is Stochastic Programming Important in Operations Research?
Operations research, or OR, is a discipline that applies mathematical and statistical methods to solve complex problems in business, engineering, healthcare, and other fields. Stochastic programming is an essential component of OR because many real-world decision problems involve uncertainty.
For example, in supply chain management, stochastic programming can help determine optimal production levels, inventory policies, and transportation schedules while considering factors such as demand volatility, transportation delays, and market fluctuations. By accounting for these uncertainties, decision-makers can make more informed and robust decisions.
Modeling with Stochastic Programming
Modeling with stochastic programming involves the following steps:
- Formulating the problem: Clearly define the decision variables, objective(s),constraints, and uncertainties.
- Specifying probability distributions: Determine the probability distributions that accurately represent the uncertainties in the problem.
- Building the mathematical model: Develop a mathematical model that incorporates the decision variables, objective(s),constraints, and probabilities.
- Solving the model: Utilize mathematical programming solvers or algorithms to solve the formulated stochastic programming model and obtain optimal solutions.
- Interpreting the results: Analyze the obtained solutions, consider their robustness, and make informed decisions based on the insights gained.
Using Springer's Resources
Springer offers a wide range of publications in operations research, including numerous books and journals dedicated to stochastic programming. These resources provide valuable insights into the theory, algorithms, and applications of stochastic programming.
Some popular books published by Springer in the field of stochastic programming include:
- "Stochastic Linear Programming: Models, Theory, and Computation" by Peter Kall and Stein Wallace
- "Stochastic Programming: The State of the Art In Honor of George B. Dantzig" edited by Stein W. Wallace and William T. Ziemba
- "Stochastic Programming: Numerical Techniques and Engineering Applications" by Andrzej Ruszczynski
In addition to books, Springer's operations research journals, such as Mathematical Programming and Computational Optimization and Applications, regularly publish articles on stochastic programming. These journals provide a platform for researchers to share their latest findings and advancements in the field.
Modeling with stochastic programming is a valuable approach for decision-makers facing uncertain environments. By incorporating randomness and uncertainty into mathematical models, stochastic programming enables optimal decision-making under risk. Springer's publications in operations research serve as excellent resources for learning and applying stochastic programming techniques. With a wide range of books and journals, researchers and practitioners can stay up to date with the latest developments in this field. Embrace the power of stochastic programming and enhance your decision-making capabilities.
4 out of 5
Language | : | English |
File size | : | 2827 KB |
Text-to-Speech | : | Enabled |
Screen Reader | : | Supported |
Enhanced typesetting | : | Enabled |
Print length | : | 97 pages |
Lending | : | Enabled |
While there are several texts on how to solve and analyze stochastic programs, this is the first text to address basic questions about how to model uncertainty, and how to reformulate a deterministic model so that it can be analyzed in a stochastic setting. This text would be suitable as a stand-alone or supplement for a second course in OR/MS or in optimization-oriented engineering disciplines where the instructor wants to explain where models come from and what the fundamental issues are.
The book is easy-to-read, highly illustrated with lots of examples and discussions. It will be suitable for graduate students and researchers working in operations research, mathematics, engineering and related departments where there is interest in learning how to model uncertainty.
Alan King is a Research Staff Member at IBM's Thomas J. Watson Research Center in New York.
Stein W. Wallace is a Professor of Operational Research at Lancaster University Management School in England.
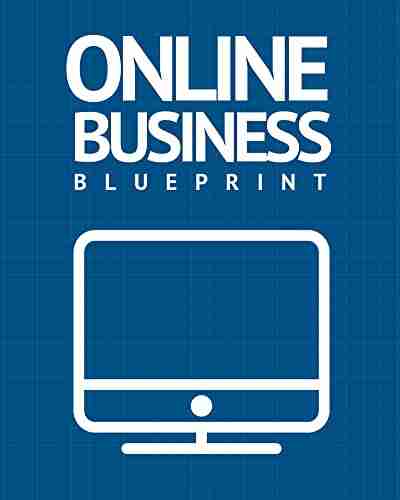

Discover the Success Story of Robert Smallwood - The...
Have you ever wondered how some...
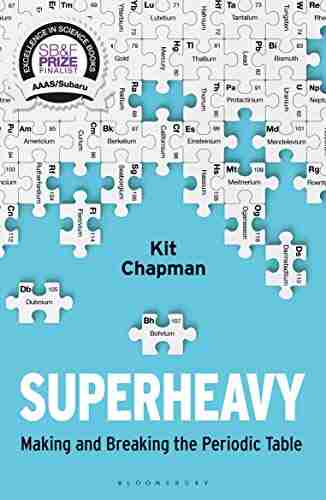

Superheavy Making And Breaking The Periodic Table
Throughout history, mankind has always...
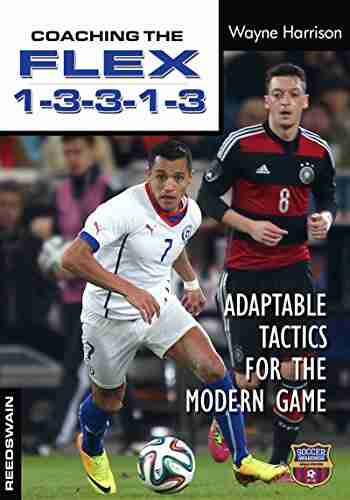

Adaptable Tactics For The Modern Game
The modern game of football is...
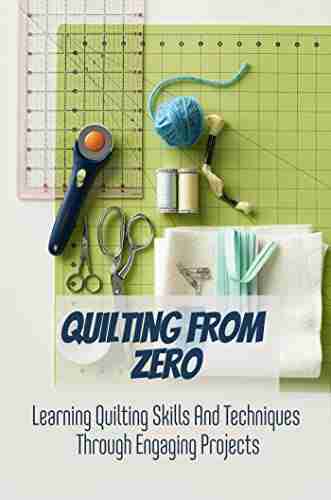

Discover the Joy of Learning Quilting Skills and...
Are you ready to embark on a...
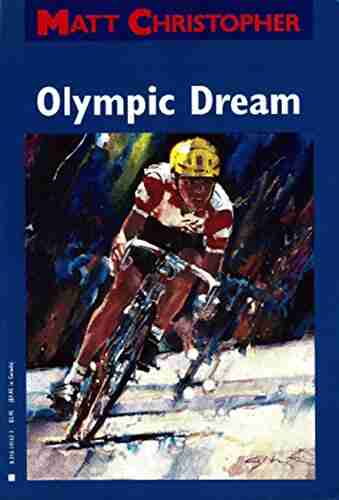

The Olympic Dream: Matt Christopher's Incredible Journey
Are you ready for an inspiring story...
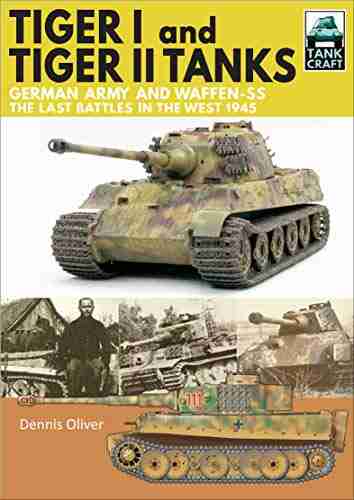

German Army And Waffen SS: The Last Battles In The West...
As history buffs and...
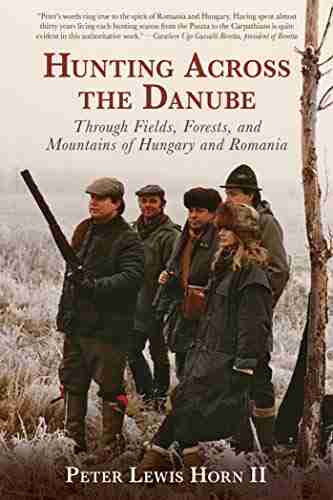

Through Fields, Forests, And Mountains: Exploring the...
Picture yourself embarking on an...
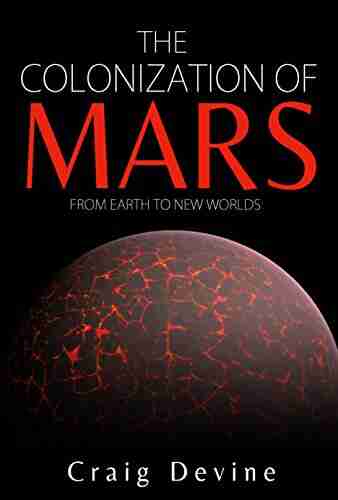

The Colonization Of Mars: A Most Mysterious Journey
Ever since the dawn of human civilization,...
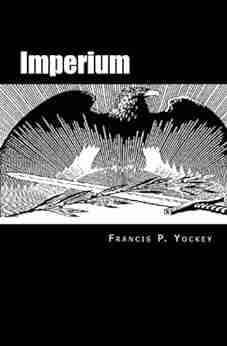

Imperium Arlie Russell Hochschild - Understanding the...
The contemporary political landscape is a...
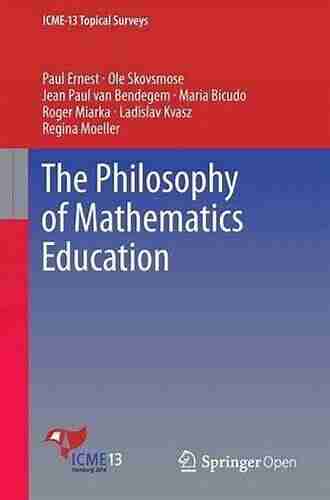

The Philosophy Of Mathematics Education Studies In...
The philosophy of mathematics education is...
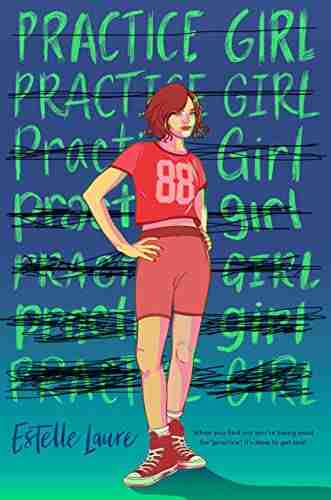

Practice Girl Estelle Laure: Unleashing Her Voice through...
Imagine a world where music is not just a...
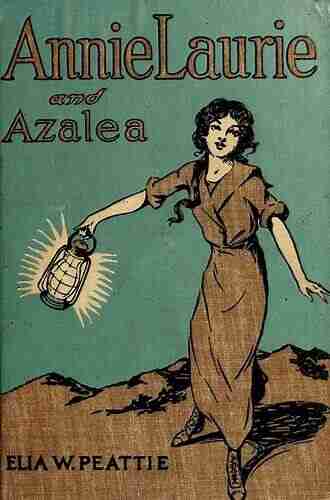

Annie Laurie And Azalea Elia Wilkinson Peattie
A Journey Through the Lives of...
Light bulbAdvertise smarter! Our strategic ad space ensures maximum exposure. Reserve your spot today!
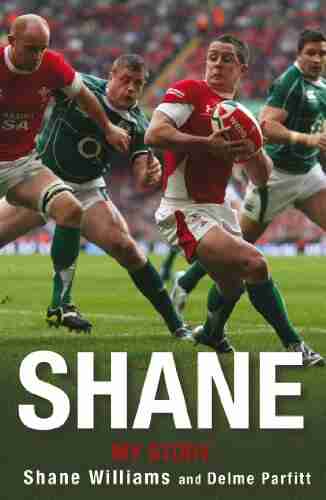

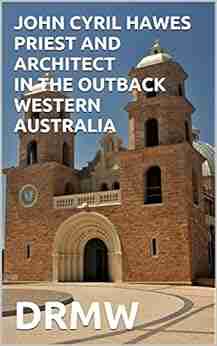

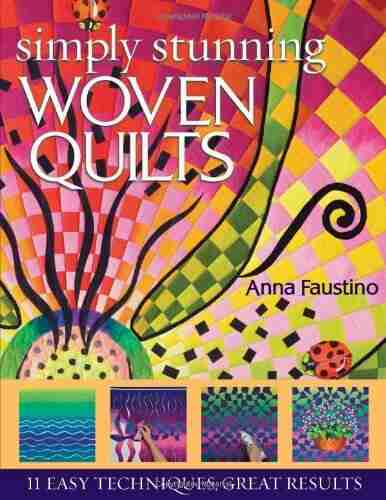

- Ralph TurnerFollow ·18.5k
- Gary ReedFollow ·7.8k
- Jon ReedFollow ·12.1k
- Asher BellFollow ·3.4k
- Tennessee WilliamsFollow ·6.2k
- Robert FrostFollow ·7.6k
- Ernest PowellFollow ·3.1k
- Terry BellFollow ·10.4k