Book Downloads Hub Reads Ebooks Online eBook Librarys Digital Books Store Download Book Pdfs Bookworm Downloads Free Books Downloads Epub Book Collection Pdf Book Vault Read and Download Books Open Source Book Library Best Book Downloads John J Macaloon Yanatha Desouvre Jason Webster Mickey Bridges Cora Leland David S Leyman John F Macarthur Rubina Ali
Do you want to contribute by writing guest posts on this blog?
Please contact us and send us a resume of previous articles that you have written.
Revolutionizing Data Analysis: Compressive Sensing and other Cutting-Edge Developments in Numerical Harmonic

Are you tired of dealing with massive amounts of data and long processing times? Do you wish there was a more efficient way to analyze and compress information? Look no further – Compressive Sensing and other developments in Numerical Harmonic have arrived to shake up the world of data analysis.
Gone are the days of traditional signal acquisition and processing methods that require a large amount of data to be sampled in order to extract meaningful information. Compressive Sensing, a revolutionary technique in the field of data science, has opened up new avenues for acquiring and analyzing data with high efficiency and accuracy, even in situations where data is scarce or restricted.
The Essence of Compressive Sensing
Compressive Sensing, also known as Compressed Sensing or CS, is a cutting-edge mathematical framework that allows the recovery of an unknown signal from a limited set of measurements or samples. Unlike traditional methods that require uniformly sampled data, Compressive Sensing entails obtaining a smaller number of measurements, while still retaining the required information for accurate reconstruction. Sounds like magic, right?
4 out of 5
Language | : | English |
File size | : | 12936 KB |
Screen Reader | : | Supported |
Print length | : | 546 pages |
At the heart of Compressive Sensing lies the concept of sparsity, which refers to the idea that most real-world signals can be represented accurately with only a few significant components. For example, an image can be sparsely represented by a small number of basis images or a specific pattern of wavelet coefficients. Compressive Sensing exploits this intrinsic sparsity to reduce the number of measurements required for signal recovery.
Applications of Compressive Sensing
The applications of Compressive Sensing are widespread and diverse, revolutionizing various fields. Let's take a closer look at a few of them:
Medical Imaging
Medical imaging techniques, such as Magnetic Resonance Imaging (MRI),often suffer from long acquisition times and high costs. Compressive Sensing has the potential to significantly speed up imaging procedures, enabling faster diagnoses and reducing patient discomfort. By exploiting sparsity in medical images, Compressive Sensing allows for accurate reconstruction from fewer measurements. This breakthrough has the potential to revolutionize the healthcare industry.
Wireless Communication
Wireless communication networks often face constraints due to limited bandwidth and battery life. Compressive Sensing offers a solution by reducing the required amount of data transmission while maintaining the integrity of the information. This technology enables efficient wireless data transfer in IoT devices, smart grids, and much more.
Big Data Analysis
The explosion of data in the digital age has posed significant challenges for traditional analytical methods. Compressive Sensing provides a powerful tool for analyzing big data by reducing the computational complexity and memory requirements. This enables faster processing and facilitates the extraction of meaningful insights from large datasets.
Other Developments in Numerical Harmonic
While Compressive Sensing has stolen the spotlight in recent years, other developments in Numerical Harmonic deserve acknowledgement. Numerical Harmonic focuses on the theory and practical applications of harmonic analysis, which deals with the representation and transformation of functions or signals in terms of harmonic functions or waves.
Among the notable developments in Numerical Harmonic is Wavelet Theory, which provides a powerful mathematical tool for analyzing signals or functions at different resolutions. Wavelets are small waves that can accurately represent various types of signals, such as audio, images, or video. The ability to analyze signals at different scales makes Wavelet Theory an invaluable asset in many fields, including data compression, image processing, and signal denoising.
Another influential development is Sparse Representation Theory, closely related to Compressive Sensing. This theory focuses on representing signals or data by using a sparse combination of elements from a given basis set. Sparse representation has found countless applications, including image restoration, speech recognition, and pattern recognition.
The Future of Compressive Sensing and Numerical Harmonic
As technology continues to advance, so does the potential of Compressive Sensing and Numerical Harmonic. These groundbreaking developments hold promise for numerous fields, from improving medical diagnoses and wireless communication to enhancing big data analytics. The ability to extract valuable information from limited measurements or representation opens up new possibilities for data analysis and compression.
So, don't wait any longer to embrace the power of Compressive Sensing and other developments in Numerical Harmonic. Stay ahead of the game and unlock the true potential of your data through these cutting-edge techniques. Prepare for a revolution in the world of data analysis!
4 out of 5
Language | : | English |
File size | : | 12936 KB |
Screen Reader | : | Supported |
Print length | : | 546 pages |
Reconstructing or approximating objects from seemingly incomplete information is a frequent challenge in mathematics, science, and engineering. A multitude of tools designed to recover hidden information are based on Shannon’s classical sampling theorem, a central pillar of Sampling Theory. The growing need to efficiently obtain precise and tailored digital representations of complex objects and phenomena requires the maturation of available tools in Sampling Theory as well as the development of complementary, novel mathematical theories. Today, research themes such as Compressed Sensing and Frame Theory re-energize the broad area of Sampling Theory. This volume illustrates the renaissance that the area of Sampling Theory is currently experiencing. It touches upon trendsetting areas such as Compressed Sensing, Finite Frames, Parametric Partial Differential Equations, Quantization, Finite Rate of Innovation, System Theory, as well as sampling in Geometry and Algebraic Topology.
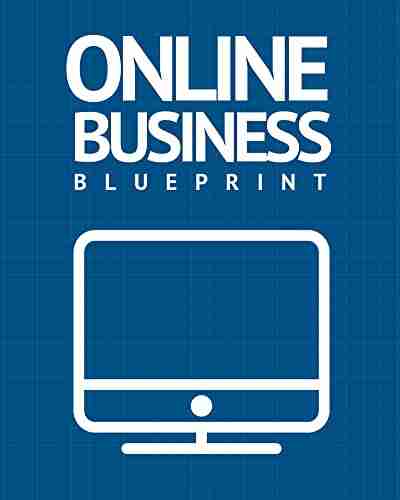

Discover the Success Story of Robert Smallwood - The...
Have you ever wondered how some...
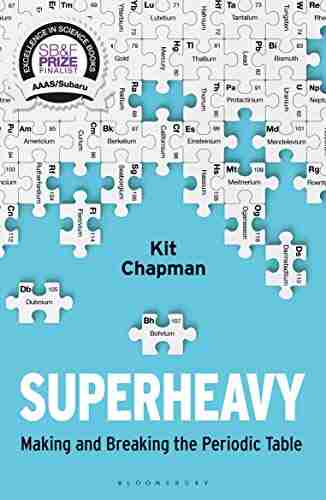

Superheavy Making And Breaking The Periodic Table
Throughout history, mankind has always...
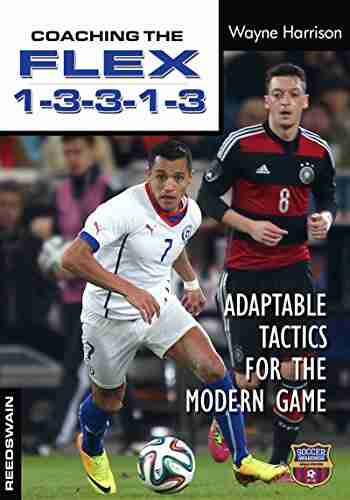

Adaptable Tactics For The Modern Game
The modern game of football is...
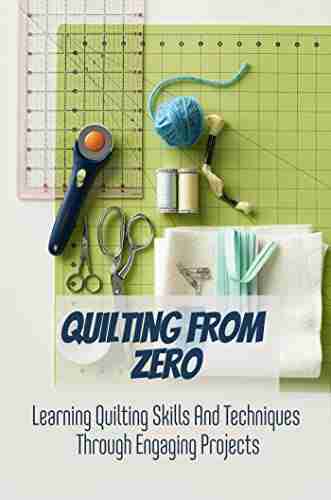

Discover the Joy of Learning Quilting Skills and...
Are you ready to embark on a...
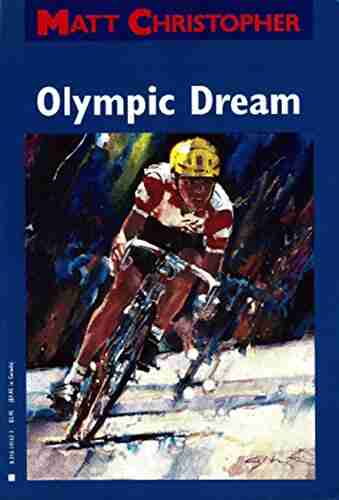

The Olympic Dream: Matt Christopher's Incredible Journey
Are you ready for an inspiring story...
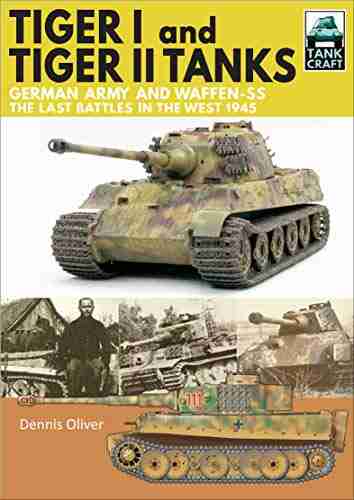

German Army And Waffen SS: The Last Battles In The West...
As history buffs and...
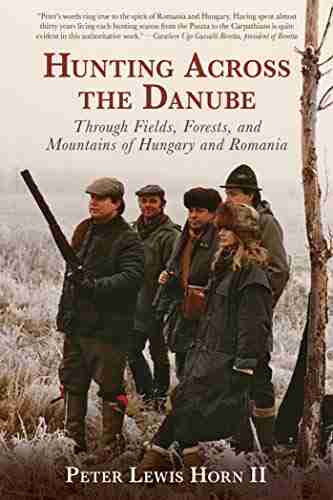

Through Fields, Forests, And Mountains: Exploring the...
Picture yourself embarking on an...
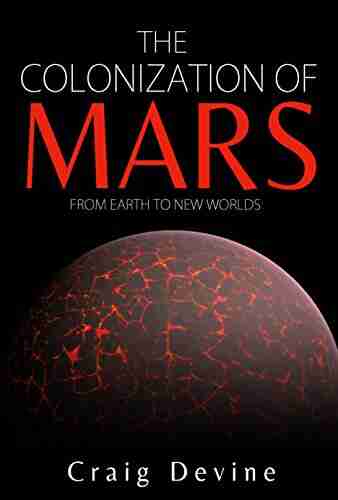

The Colonization Of Mars: A Most Mysterious Journey
Ever since the dawn of human civilization,...
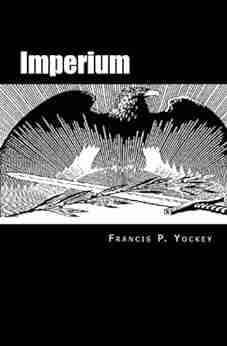

Imperium Arlie Russell Hochschild - Understanding the...
The contemporary political landscape is a...
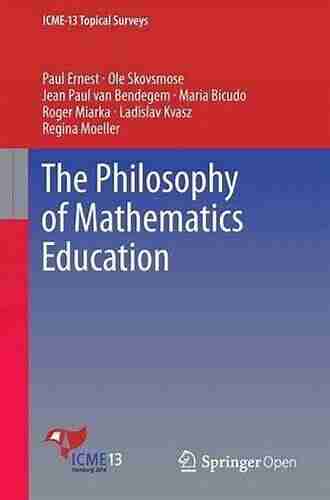

The Philosophy Of Mathematics Education Studies In...
The philosophy of mathematics education is...
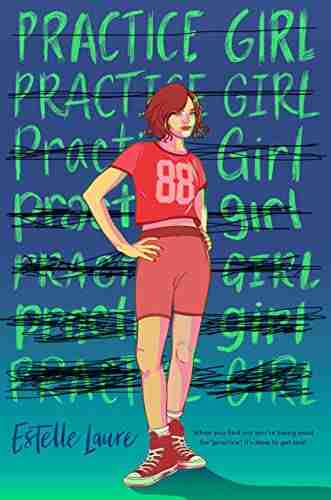

Practice Girl Estelle Laure: Unleashing Her Voice through...
Imagine a world where music is not just a...
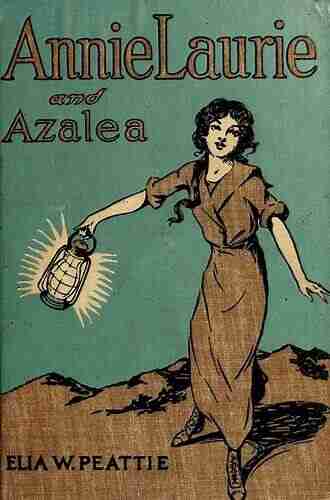

Annie Laurie And Azalea Elia Wilkinson Peattie
A Journey Through the Lives of...
Light bulbAdvertise smarter! Our strategic ad space ensures maximum exposure. Reserve your spot today!
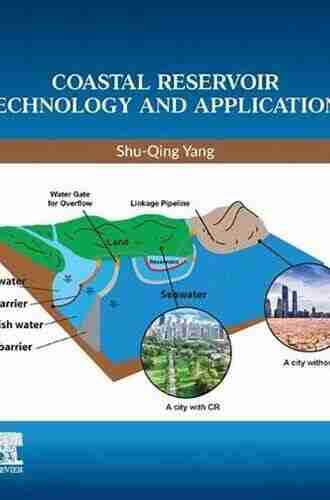

- Corey GreenFollow ·5.4k
- Giovanni MitchellFollow ·10.3k
- Nikolai GogolFollow ·3.6k
- Levi PowellFollow ·12.1k
- Ruben CoxFollow ·6.5k
- Jason HayesFollow ·10.3k
- Harrison BlairFollow ·5.6k
- Timothy WardFollow ·7.1k